TL;DR
This newsletter is about whether large language models (LLMs) store personal data they may have been trained on. It looks at how LLMs work, the definition of personal data under the GDPR and the various arguments around this issue.
Here are the key takeaways:
In July 2024, the Hamburg DPA published a discussion paper arguing that LLMs do not store personal data. That paper contends that LLMs only store the correlations between fragments of words (i.e., tokens) in a numerical form, which does not constitute information that can be used to identify a person.
The Hamburg DPA explains how the complex architecture of LLMs transforms personal data into these numerical representations. Such architectures process its training data in such a way that the information stored by the LLM on its own cannot be linked back to specific individuals.
However, if the model comes across certain data in its training data frequently enough, it can create strong correlations between the tokens that make up that data. Those strong correlations may be learned and retained by the model such that the correct prompt can be used to extract this data.
LLMs may therefore be regarded as storing personal data that it has memorised in this way. Accordingly, such personal data would constitute pseudonomized data when stored in the model, and then becomes readable when the correct prompt is used to generate it from the model.
European data protection authorities appear to have diverging views on this issue. This could be addressed by the European Data Protection Board in a future opinion it may publish on AI models and the GDPR.
Summary of the Hamburg paper
Back in July, the Hamburg data protection authority (DPA) published a discussion paper looking at whether LLMs store personal data.
The argument presented by the Hamburg DPA in their paper is as follows:
The text data collected for the training of the LLM (which may contain personal data) go through a process of tokenisation. With this process, the text data are broken up into chunks (either individual words or fragments of words) and converted into numerical values (i.e., tokens).1
These tokens are then processed into word embeddings. These embeddings consist of information about the correlations between the different tokens.2
LLMs do not store whole words or sentences. Instead, they only store "linguistic fragments" as "abstract mathematical representations" and learn the correlations and patterns between these fragments during its training as contained in its parameters.3
So when an LLM produces its output, it uses the correlations and patterns contained in its parameters to combine the different fragments together. This is used to produce whole sentences and paragraphs in a response to a given prompt.
Accordingly, LLMs produce probabilistic outputs (i.e., the outputs are predictions of what it thinks the best response to the prompt is based on the learning obtained from its training). This is different from deterministic outputs that a database query would return from a database, where a certain input always produces the same output.4
Based on this argument, the Hamburg DPA concludes that LLMs do not store personal data as per the GDPR.
The paper distinguishes the information stored in LLMs from "IP addresses, exam responses, legal memos by public offices, vehicle identification numbers or other coded character strings such as the [transparency and control] string."5 In doing so, it cites the different CJEU cases touching on the processing of each type of personal data.
The common thread running through all these cases is that these types of data "indicate a reference to the identification of a specific person or to objects assigned to persons".6 This makes such data "identifiers" or information "relating" to a data subject.
This stems from the function that such data performs. For example, the purpose of an IP address is to specify the location of an internet-enabled device so that it can receive and send information via the internet.
The tokens stored in LLMs, on the other hand, "lack individual information content and do not function as placeholders for such."7 The models only store "highly abstracted and aggregated data points from training data and their relationships to each other, without concrete characteristics or references that "relate" to individuals."8
Even the existence of LLM privacy attacks do not change this finding. I have written previously on how OpenAI's GPT models can be subject to training data extraction attacks.
The Hamburg DPA contends that such attacks do not evidence the storage of personal data in LLMs for two main reasons:
The presence of personal data in an LLM's output "is not conclusive evidence that personal data has been memorized, as LLMs are capable of generating texts that coincidentally matches training data."9
As per CJEU case law, "data can only be classified as personal data if identification is possible through means of the controller or third parties that are not prohibited by law and do not require disproportionate effort in terms of time, cost and manpower."10
The implication of the Hamburg's arguments is that, if an organisation takes a copy of an LLM produced by a developer, the mere hosting of that LLM in its own environment does not mean that it is storing the personal data the model may have been trained on. All that is contained in the model are tokens, not personal data.
But I think the other more significant implication relates to data subjects rights, which the Hamburg notes in its paper:
A person enters their name into a company's or authority's LLM-based chatbot. The LLM-based chatbot provides incorrect information about them. Which data subject rights can they assert in relation to which subject matter?
As LLMs don't store personal data, they can't be the direct subject of data subject rights under articles 12 et seq. GDPR. However, when an AI system processes personal data, particularly in its outputs or database queries, the controller must fulfil data subject rights.
In the case outlined above, this means that the data subject can request the organization to provide, at least regarding the input and the output of the LLM chatbot,
that information is provided in accordance with article 15 GDPR,
that their personal data is rectified in accordance with article 16 GDPR,
if applicable, that their personal data is erased in accordance with article 17 GDPR.11
To be clear, the Hamburg DPA does still argue that personal data may be contained in the training data used to develop the LLM. But after that training data has been used for this purpose, the resulting trained model does not itself store that personal data.
Accordingly, it is implied that organisations wanting to fine-tune LLMs in their own environment are exempt from certain GDPR obligations, such as data subjects requesting the rectification of their personal data in the model.
How do LLMs work?
There are many different LLMs out there at this point. This post just focuses on the GPT models by OpenAI, although generally other LLMs work in a similar way.
The GPT model consists of the following components:
Tokenisation
Word embeddings
Positional encoding
Transformer blocks
Probability distributions
Fine-tuning
Tokenisation
For LLMs to work with a text input, it needs that text to be converted into a string of numbers to interpret and perform functions on them.
This is what tokenisation is about. When an LLM takes in text data as an input, it converts that data into a string of numbers using a tokeniser.
This is a mechanism that "splits the text into a vocabulary of smaller constituent units (tokens) that can be processed by the [LLM]."12 This vocabulary consists of "both common words and word fragments from which larger and less frequent words can be composed."13
For example, if we took OpenAI's tiktoken, which is a tokeniser that is uses for its GPT models, and applied it to the sentence "With great power comes great responsibility", each word in that sentence would be represented as values that would look like the following:
[2886, 2212, 3470, 5124, 2212, 16143]
Accordingly, from the perspective of the LLM:
"With" turns into
2886
"great" turns into
2212
"power" turns into
3470
"comes" turns into
5124
"responsibility" turns into
16143
As the Hamburg DPA points out in is paper though, the tokenisation for LLMs will convert both whole words and fragments of words into tokens. So in reality tokenising the above sentence would result in more tokens representing fragments of the words contained in that sentence.
Word embeddings
After tokenisation, the tokens go through an embedding layer whereby the tokens are mapped to a word embedding.
The purpose of this is to identify the relationships between the tokens. This helps to separate tokens that are closely related to each other from those tokens that are not related to each other.
The embedding layer encodes each token in a matrix so that each token represents a vector. In the context of LLMs, a vector is essentially a list of numbers whereby each value is 0
except for the value corresponding to the token which is set to 1
.14
For example, the vectors for the tokens in the sentence "With great power comes great responsibility" would look something like the following:
[[1. 0. 0. 0. 0. 0.]
[0. 1. 0. 0. 0. 0.]
[0. 0. 1. 0. 0. 0.]
[0. 0. 0. 1. 0. 0.]
[0. 1. 0. 0. 0. 0.]
[0. 0. 0. 0. 1. 0.]]
Above is 2-dimensional array (or matrix) where each row represents a word in the sentence. For example, the first row [1. 0. 0. 0. 0. 0.]
represents the word 'With'. The row [0. 1. 0. 0. 0. 0.]
represents 'great' and appears twice in the matrix since that word appears twice in the sentence.
When these embeddings are projected onto a graph, you can visualise how close or far away the tokens are from each other. The screenshot below is from 3Blue1Brown's video on YouTube on LLMs that shows what these word embeddings look like:
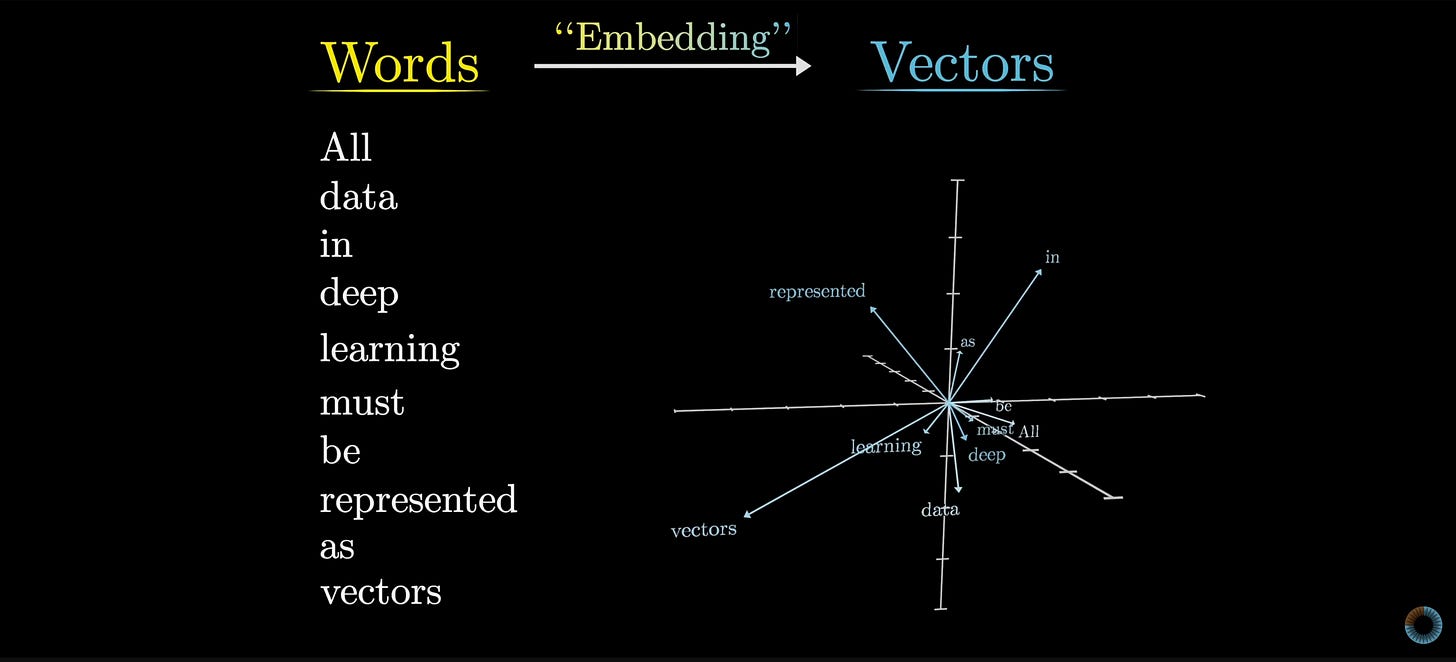
In the screenshot you can see the connections the LLM picks up from the sentence "All data in deep learning must be represented as vectors" by looking at where it places each word from that sentence on the graph. However, the screenshot only shows the vectors projected onto a geometric space with a few dimensions.
For LLMs, the vectors for the word embeddings will have thousands of dimensions that capture several different relationships between the tokens. For example, GPT-3 processes word embeddings with over 12,000 dimensions.15
Positional encoding
Following the embedding layer is the positional encoding mechanism. The purpose of positional encoding is to identify the position of each word in the sentence and capture this information within a vector.
This enables the LLM to have a better understanding of the text data it processes, including grammar and syntax. The vector representing the positional encoding of a word in a sentence might look something like this:
[5.40302306e-01 3.87674234e-01 9.87466836e-01 6.30538780e-02 9.99684538e-01 9.99983333e-03 9.99992076e-01 1.58489253e-03 9.99999801e-01 2.51188641e-04 9.99999995e-01 3.98107170e-05 1.00000000e+00 6.30957344e-06 1.00000000e+00 1.00000000e-06 1.00000000e+00 1.58489319e-07 1.00000000e+00 2.51188643e-08]
Transformer blocks
After the text data goes through the tokeniser, embedding layer and positional encoder of the LLM, it is transformed into a new input comprising of three sets of information: the token, the embedding vector and the positional encoding vector.
This input then goes through the transformer blocks. This is the crux of the LLM.
The transformers used for the GPT models consist of what are known as decoders. These decoders consist of multiple layers of (i) masked self-attention mechanisms, and (ii) feed forward neural networks.
Attention is essentially about looking at every word in the given text data to understand the relevant context. But the GPT models use a specific technique called self-attention, where the model looks only at the words in the given input text data to gauge the correct context that in turn helps to identify the correct meaning of the sentence.
Take the following two sentences:
"Server, can I have the check please?"
"Looks like I just crashed the server."
Both sentences include the word 'server'. However, that word has a different meaning in each sentence. The way that we can tell this is by observing the other words in the sentence.
In the first sentence, the word 'check' indicates that the type of server being used in that sentence describes an employee in a restaurant. In the second sentence, the word 'crashed' indicates that the server being referred to is a type of computer.
This is what self-attention is about; the model is observing the words in the given text data input to identify the relevant context and therefore interpret the sentence correctly. However, the GPT models add one more step to this, which is where the 'masking' comes in:16
When training the model, we could feed it the whole sentence, for example: "With great power comes great responsibility."
However, the GPT models are autoregressive, meaning that the LLM is trained to generate an output word-by-word, where the predicted next word is conditioned on the previous words.
Accordingly, if we gave the model the full sentence, it would essentially see the answers that it is supposed to be predicting and the model would not be learning properly.
So instead of instructing the model to look at all the words in the sentence, we can instruct the model to only look at the current or previous words.
For example, we would give the model the words "With great power comes" so that it can apply the self-attention mechanism to those words in order to predict the next word, which in this case the correct next word would be "great".
The masked self-attention mechanism ultimately gives each word in the input a score that essentially represents the level of attention that each word should be given. After being assigned a masked self-attention score, the text data then flows through a feed forward neural network.
The role of the neural network is essentially to find a path from the input text data to the correct next word in the sentence. This is done by activating the correct neurons in each layer in the network and applying the correct values for the weights and bias (i.e., the parameters of the neural network).
The network learns to find these pathways by:
Making a prediction on the input
Measuring the distance between the prediction and the true output using a loss function and recording this within a loss score
Feeding the loss score into an optimizer that then adjusts the parameters based on this loss score (using a process called gradient descent)
The ultimate goal of all this is to find the optimal set of parameters that make the most accurate predictions. The GPT models are trained to do this in an unsupervised manner, as Stephen Wolfram explains in his blog post:
Recall that the basic task for ChatGPT is to figure out how to continue a piece of text that it’s been given. So to get it “training examples” all one has to do is get a piece of text, and mask out the end of it, and then use this as the “input to train from”—with the “output” being the complete, unmasked piece of text...the main point is that—unlike, say, for learning what’s in images—there’s no “explicit tagging” needed; ChatGPT can in effect just learn directly from whatever examples of text it’s given.
Probability distributions
After the text data in a prompt goes through the several layers of decoder blocks in the transformer architecture, the LLM will map each word embedding to all the tokens in its vocabulary (i.e., all the tokens that the model has learned from its training data).17 A function is then applied to convert the mappings into probabilities for each word.
What the model is doing here is looking at all the tokens that it knows and working out the probability of each of token being the next word in the given input text data. This is what is meant by a probability distribution.
For example, if we provided the model with the words "With great power comes", it might produce the following probability distribution:
Top 10 predicted words:
great: 0.24902514
with: 0.0548905
a: 0.0316601
in: 0.02712334
after: 0.025105478
lies: 0.02287713
to: 0.020820534
that: 0.016439462
and: 0.015829766
an: 0.01466654
At this point, there are a few different ways to select a word from this probability distribution. The simplest method would be to select the word with the highest probability.
Alternatively, to make the LLM generate more nuanced outputs, you could instruct the model to select from a sample of the distribution. For example, with top-k sampling, the model will select the next word "from only the top-K most likely possibilities."18
If k is set to 10, let's say, then the model will randomly select from one of the 10 most probable words from the probability distribution. The purpose of using a method like top-k sampling is to prevent the model "from accidentally choosing from the long tail of low probability tokens and leading to an unnecessary linguistic dead end."19
How LLMs generate text
Putting all the above together, the GPT models generate new text in the following way:20
A tokeniser is applied to the input text data to convert the natural language into tokens (i.e., a string of numbers).
The tokens go through an embedding layer where each token is mapped to a word embedding, resulting a matrix of vectors where each vector represents each token.
A positional encoder adds another vector to each word in the input text data representing the position of word in the sentence.
These vectors then go through multiple layers of decoders in a transformer architecture consisting of a masked self-attention mechanism and a feed forward neural network:
The masked self-attention mechanism gives each token a score representing how much attention should be given to that word in the context of the input.
The feed forward neural network finds the best path between the tokens and the correct next word in the sentence.
The model produces a probability distribution and selects a token from this distribution.
But in order for the model to produce longer bodies of text, there are two more steps involved:
6. The model adds the predicted token to the original input to create a new input.
7. The LLM then takes in the new input and repeats steps 1-6 until it creates a complete sentence or even a paragraph.
Fine-tuning
I won't go into how fine-tuning works since it is not too pertinent to the topic of this post. However you can read my previous explanations of fine-tuning, both a simple explanation in a post on OpenAI's o1 model and a more detailed explanation in a post on Google's Gemini model.
What constitutes the 'procesing' of 'personal data' under the GDPR?
Under Article 4.1 of the GDPR, 'personal data' is defined as:
any information relating to an identified or identifiable natural person (‘data subject’); an identifiable natural person is one who can be identified, directly or indirectly, in particular by reference to an identifier such as a name, an identification number, location data, an online identifier or to one or more factors specific to the physical, physiological, genetic, mental, economic, cultural or social identity of that natural person.
This definition can be broken down into four main elements:
'any information'
'relating to'
'identified or identifiable'
'natural person'
'any information'
Personal data can include information that may be objective or subjective in nature. This means that opinions or assessments about a person can constitute their personal data.21
The content of personal data could include information about one's private life that could be regarded as sensitive, but it is not just limited to this.22 Recital (30) GDPR states that online identifiers, such as IP addresses and cookie identifiers, can also be classed as personal data.
This information can also come in a variety of forms. It could be in a written form or even a sound or image.23
'relating to'
For information to be personal data under the GDPR, that information must be about an individual. This means that either the content, purpose or effect must be linked to a particular person:24
The content element is satisfied if the information itself is about an individual, such as the exam result of a student.
The purpose element is satisfied if the information can be used to evaluate or analyse an individual.
The effect element is satisfied if the use of the information has an impact on an individual's rights or interests.
'identified or identifiable'
Under the definition of personal data, identifiability means a data controller or another person being able to single out an individual either directly or indirectly.
Whether a piece of information can be used to identify a person must be assessed from the perspective of those parties with access to that information.25 This could be the data controller or a third party that manages to gain access to the data.
However, Recital (26) states that account must be taken of "means reasonably likely to be used" to identify a person:
To ascertain whether means are reasonably likely to be used to identify the natural person, account should be taken of all objective factors, such as the costs of and the amount of time required for identification, taking into consideration the available technology at the time of the processing and technological developments.
Accordingly, the CJEU has held that:
...if the identification of the data subject was prohibited by law or practically impossible on account of the fact that it requires a disproportionate effort in terms of time, cost and man-power...the risk of identification appears in reality to be insignificant.26
'natural person'
To be personal data, the information must relate to a natural person, and therefore not corporations, partnerships or other legal persons. Additionally, Recital (27) states that the GDPR does not apply to deceased persons.
Processing of personal data
Under Article 4.2 GDPR, the 'processing' of personal data means any operation performed on that data. This includes storing personal data, but also includes, among other things, its collection, organising, structuring, alteration, transmission or dissemination.
Furthermore, the CJEU has previously found that processing:
...may consist in one or a number of operations, each of which relates to one of the different stages that the processing of personal data may involve.27
Keep reading with a 7-day free trial
Subscribe to The Cyber Solicitor to keep reading this post and get 7 days of free access to the full post archives.