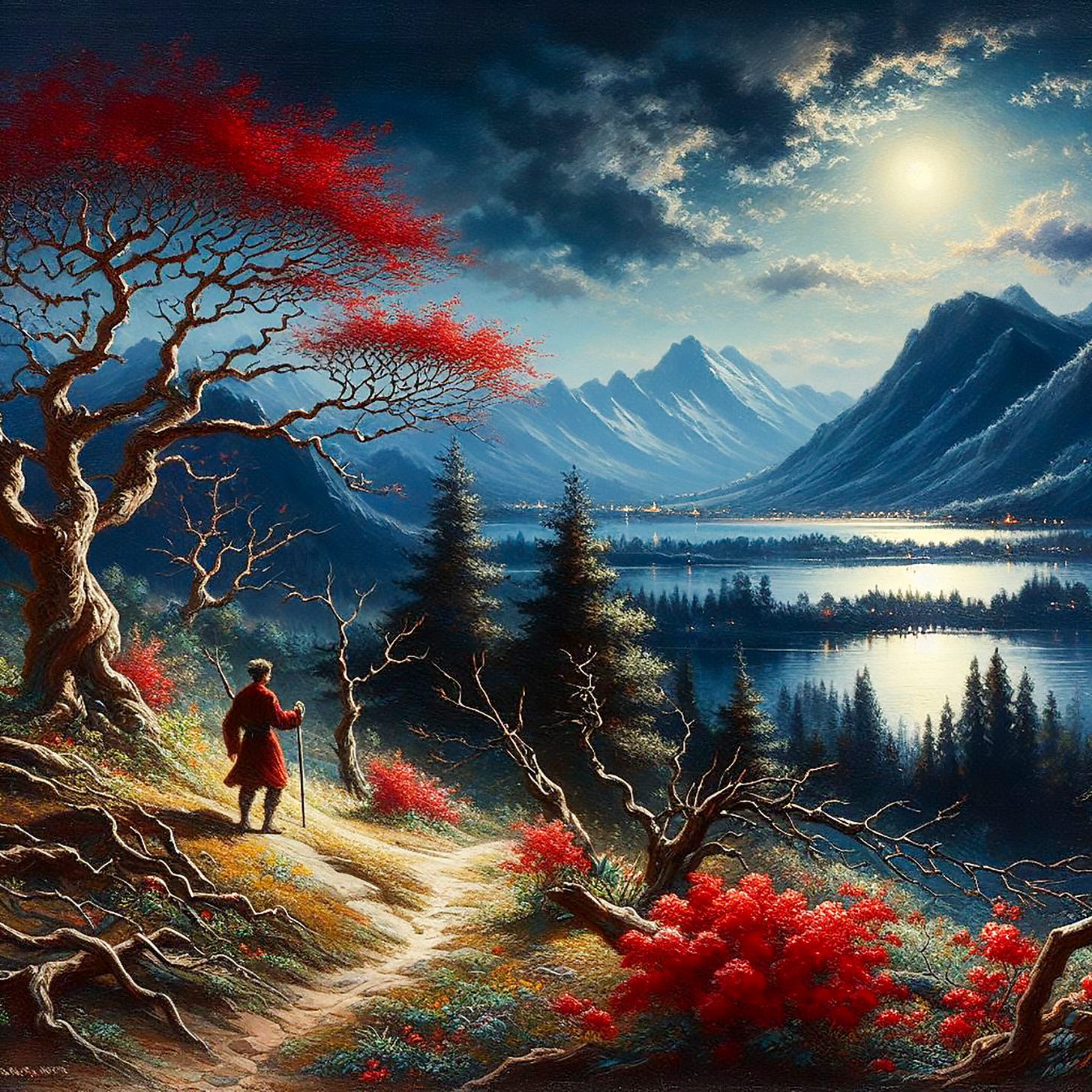
The data-hungry generative models dominating the current AI hype are having stark negative impacts on data rights.
Constructing the training datasets relies on two main data acquisition methods. One is scraping data from the internet, and the other is repurposing user data previously collected for other purposes.1
But the current AI hype is encouraging bad practices around these data acquisition methods.
Only a few days before its IPO, Reddit revealed that it was being investigated by the US Federal Trade Commission (FTC) for the company's deals to provide user data for AI model development. Reddit contends that it has not "engaged in any unfair or deceptive trade practice".
Automattic, the company behind platforms like Tumblr and WordPress, has also been entering into deals to sell user data (including blogposts, comments and articles) to OpenAI and Midjourney. It has stated that it will share "public content that's hosted on WordPress.com and Tumblr from sites that haven't opted out."
Plus, as I have written about previously, Meta has been using data from its users to develop its AI models. This includes millions of images from Instagram and Facebook.
Steph Ango, the current CEO of Obsidian (my favourite writing app), has compiled a list on X (formerly Twitter) of other companies that have taken similar routes. This includes Google, Zoom and Dropbox.
And to top it all off, during a recent interview with The Wall Street Journal, Mira Murati, the current CTO of OpenAI, was asked what data its video generation model Sora was trained on. This is what she said:
Developers constructing web-scraped datasets for model development implicitly support the idea that just because data is public, it can be used for any purpose they want. This idea is flawed:
Even though data might be exposed to the public, there can still be privacy interest in the data because it is hard to find, not normally observed or recorded, and fragmented and widely dispersed.2
Similarly, developers repurposing user data for model development show a disregard for transparency and data subjects having control over their data. As Ango puts it:
if your data is stored in a database that a company can freely read and access (i.e. not end-to-end encrypted), the company will eventually update their ToS so they can use your data for AI training — the incentives are too strong to resist
You could say that a lot of this activity is being driven by two factors:
The scaling doctrine. In ML research, training really big models on massive training datasets is touted as the clearest path to optimal performance. Collecting as much data as possible is a crucial part of this.
Profit incentives. Developers must grow as fast as possible in the pursuit of profits. This is what the venture capitalists backing them are encouraging.
There are three data protection principles which, if followed, would help tame the data-hungry practices being spurred on by AI hype:
Lawfulness, transparency and fairness. This requires a legal basis for the use of the data, that its use is made known to data subjects, and that data should not be collected by deception or without the knowledge of the data subject.
Purpose limitation. This requires that data are only used for specified, explicit and legitimate purposes. It also cannot be used for other purposes unless they are compatible with the original purpose.
Data minimisation. The data used should be adequate, relevant and limited to what is necessary for the purpose of its use.
If these principles were followed, AI developers would be:
Justifying their use of data
Notifying data subjects before using their data
Minimising the amount of data used to that which is actually necessary
Let's see if this ever happens.
Daniel J. Solove, ‘Artificial Intelligence and Privacy’ (Draft, March 2024), p.23.
Daniel J. Solove, ‘Artificial Intelligence and Privacy’ (Draft, March 2024), p.25.