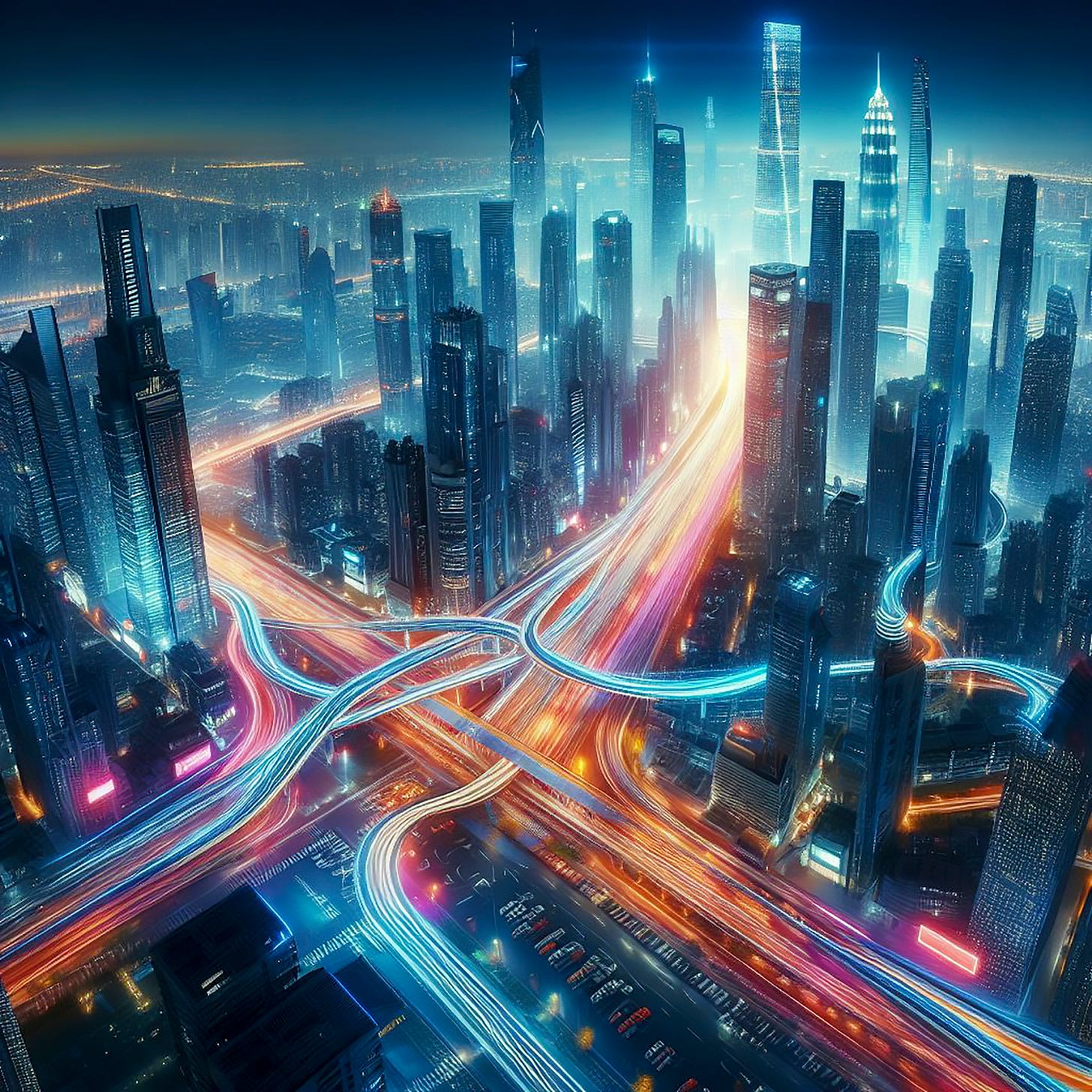
I recently read a thesis on AI by Abeba Birhane, a cognitive scientist researching human behaviour, social systems, and responsible AI. Her thesis is called Automating Ambiguity: Challenges and Pitfalls of Artificial Intelligence.
It is a fascinating read that challenges AI hype in ways that I have not previously considered. And there was one criticism highlighted by Birhane that I think is particularly thought-provoking.
AI research, in its current form, encourages stagnation rather than progress.
This is how Birhane puts it:
Predictive models, due to their use of historical data, are inherently conservative. They reproduce and reinforce norms, practices, and traditions of the past.1
Humans, and the societies we live in, are inherently complex. This implies non-stationary entities that cannot be "captured in neat taxonomies" and instead "are active, dynamic, historical, social, cultural, gendered, politicized, and contextualized organisms."2
Accordingly, "humans beings and their behaviour are complex adaptive phenomena whose precise pathway is simply unpredictable."3 Attempting to precisely condense this complexity into easily measurable and interpretable models is incredibly difficult, if not impossible.
But this is exactly what ML is intended to do. ML models are tools for prediction that rely on statistical correlations and "process data that supposedly capture people's behaviours, actions, and the social world, at large."4
However, in trying to model and predict the world, ML ends up reinforcing the correlations and patterns it identifies. As Birhane contends, ML systems "force order, equilibrium, and stability to the active, fluid, messy, and unpredictable nature of human behaviour and the social world at large."5
So in a way, ML models become self-fulfilling prophecies. Their outputs "are used to justify action in the social world and actions in the social world are datafied and fed into models."6
Accordingly, ML systems end up maintaining the very social orders that they were merely designed to model and predict. They reinforce what they observe via one big feedback loop.
It is this idea that forms one of the central themes of Birhane's thesis:
The practice of constructing predictive models based on the past and directly deploying them for decision-making amounts to constructing a programmed vision of the future based on an unjust and socially conservative past.7
On this view, AI may not after all be the key to progression, as some propose. Instead, in the long-run, AI might just end up preserving what already is, leaving us in a undesirable state of stagnation.
Abeba Birhane, Automating Ambiguity: Challenges and Pitfalls of Artificial Intelligence (2022), p.42.
Abeba Birhane, Automating Ambiguity: Challenges and Pitfalls of Artificial Intelligence(2022), p.36.
Abeba Birhane, Automating Ambiguity: Challenges and Pitfalls of Artificial Intelligence (2022), p.30.
Abeba Birhane, Automating Ambiguity: Challenges and Pitfalls of Artificial Intelligence (2022), p.34.
Abeba Birhane, Automating Ambiguity: Challenges and Pitfalls of Artificial Intelligence (2022), p.28.
Abeba Birhane, Automating Ambiguity: Challenges and Pitfalls of Artificial Intelligence (2022), p.39.
Abeba Birhane, Automating Ambiguity: Challenges and Pitfalls of Artificial Intelligence (2022), p.42.
"But this is exactly what ML is intended to do. ML models are tools for prediction that rely on statistical correlations and "process data that supposedly capture people's behaviours, actions, and the social world, at large."
I don't know if I entirely agree. I don't think all ML models are designed to be tools for prediction, or 'capture people's behaviours, actions, and the social world'. There are plenty of uses for ML that don't involve any of that (generative ML models, summarization, processing large datasets to look for anomalies, generation of novel structures, proteins, techniques, conversation)
None of those prediction or capturing people's behaviours, etc. So, Birhane is right in the sense that use of AI/ML models for such things would be a net-bad and would, in fact, stagnate society. In some respects, that's what @desystemize was on about as well. That is one area where I think law can be effective (using AI for any sort of prediction with legal or similarly significant effects should be prohibited, full stop).
But I do think it's important to remember nuance and that one terrible use of ML is not necessarily indicative of all uses.
"ML systems end up maintaining the very social orders that they were merely designed to model and predict. They reinforce what they observe via one big feedback loop."
This is such an important point. LLMs and generative AI are based on data from the past and cannot easily adapt to new data. Thus they keep reinforcing stereotypes, social orders, mainstream opinions, etc. in an eternal feedback loop to the detriment of culture .
I am currently reading "Filterworld: How Algorithms Flattened Culture" by Kyle Chayka where he makes the same point about recommendation algorithms.